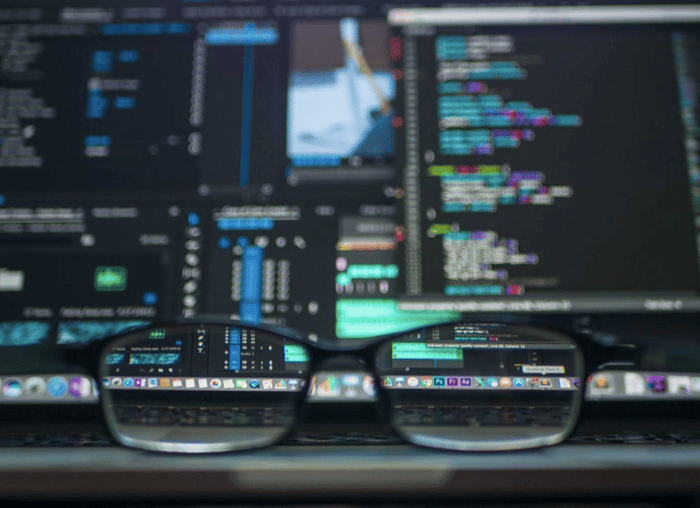
Evolving into a data-driven organization has gone mainstream over the past 10 years. Roughly half of companies say they’re using data to drive strategy, and most admit to stockpiling data and reporting on it. In fact, it’s almost become difficult to get away from data.
Nearly every application comes with an analytics tab. Implementing tracking on products, campaigns, processes and digital experiences has become easy as copy-paste. Our iPhones even report back to us how much time we spend on them.
But success doesn’t come from looking at data; it comes from failure — and using data to experiment until you get it right.
Data won’t protect you from failure
When something doesn’t pan out, data won’t tell you what the pitfall is — or how to get over it.
Yet, it will call your attention to a specific area, pointing to it like a blinking neon sign that says, “look here!” And that’s when you take the reigns and try something new. Data can also tell you whether your solutions are working (the blinking neon sign then reads, “getting warmer!”) and when you’ve taken a turn toward getting it right. This is called experimentation.
Experimentation isn’t a shot in the dark
As Google’s global head of customer analytics Neil Hoyne points out, time and again, companies make “crushingly common mistakes with data, and refuse to give themselves the room to experiment and to fail.”
For data-driven organizations, it’s a required skill set to turn the data you see into lessons you learn. It’s a fast-moving discipline that requires hands-on attention and a full-court press.
While different practices, from UX to product development, ops to growth marketing, have their nuanced approaches to experimentation, we’ve outlined a straightforward framework to help your organization harness the power of data with true experimentation.
7-Phase Experimentation Cycle
1. Goal
Set one. Be smart about it: make is specific, measurable, achievable, realistic and time-restrained. What do you want users to do — or what should a new process achieve? How will you know you’ve done it? Is it even possible? Be mindful of how big a problem you’re trying to solve, and whether you’ll learn more, fail faster if you break it down into components.
2. Paired Indicators
Dig deep to identify the metrics that not only spell out what success looks like, but mind the consequences of what you do to achieve your goals. For example: you set a record for support tickets resolved, but it’s at the expense of customer satisfaction. You don’t want to meet your goal and sink the rest of the company.
3. Timeframe
Your goal will make it clear how long you have to meet it. But setting interval timeframes or sprints can help you incrementally work, measure and tweak your way to that goal.
4. Starting point
Options abound for where to start working toward your goal. Pick one. Identify your opportunities. Consider where you have data — and where that data is blinking its biggest and boldest signs. Where is your data saying, “look here!” Look there.
5. Hypothesis
Create a solution to test and verify — a prediction of what will happen if you try (this). Get your team to agree to it. It’s your hypothesis. In Designing With Data, Elizabeth Churchill et al. prescribed a helpful format for data-driven hypotheses (which can be adjusted and used for your project or line of work):
For [user group], if [change] then [effect] because [rationale], which will impact [measure].
Here are a few examples of well-formed hypotheses.
6. Test + Results
Finally, a test that’s equally good if you succeed and if you fail. Here’s why: if you succeed, you’ve succeeded! Your solution has done what it was supposed to (also, pat yourself on the back — you were right!).
If you fail, you have the opportunity to find out why. Go back into your data and look for those blinking signs. A few questions to consider:
- What changed when you put your solution to work?
- What does your data tell you the solution did that you hadn’t anticipated?
- What does your data tell you the solution didn’t do that it should have?
- What parts of your solution worked to change results, even a little?
- How might human behavior (and its unpredictability) have affected the outcome?
7. Iterate
Take the results from your test(s). Reform your hypothesis. Lather, rinse, repeat until you’ve learned something. Test it. Get the results you’ve been looking for.
An experimental example
Keep in mind, when we say “solution,” it doesn’t simply apply to technology solutions. It can. But it can also be testing new email campaigns or website designs, better open enrollment processes or troubleshooting inefficiencies in accounts receivable.
Consider this example: Testing a Solution for Better New Employee Onboarding
- Goal: Increase completion rate of new-employee onboarding experience by 15% over the next 2 quarters
- Paired Indicator: Productivity lost by HR, managers, support staff
- Timeframe: With a rate of 25 new employees onboarded each month, we’ll check-in, measure and tweak every two weeks for the quarter.
- Starting Point: Provide a daily agenda that sets clear expectations for completion of tasks and paperwork across the first 90 days of employment.
- Hypothesis: For new employees, if agendas outline steps and deadlines for onboarding work to be completed then new employees will be more likely to complete tasks, on time, because HR and hiring managers will have set clear performance expectations, which will impact the new employees’ assimilation into the company and ability to start their new jobs with a solid foundation — and benefits.
- Test: HR works with hiring managers to create department-specific daily agendas for new employees to follow for the first 90 days of onboarding. These will be printed on paper and given to employees each day.
- Results: More tasks are getting done on time for the first 14 days, but falling off. Paper agendas aren’t getting printed. Hiring managers are forgetting to give them to new employees. Papers are getting lost.
- Iterate: Agendas seemed to work. They set clear expectations for what needed to be done, when. But they didn’t keep employees engaged. Next test: move these agendas and checklists into Trello and use date reminders.
It doesn’t need to be big, just forward
Experiments don’t need to be big to be successful — or a well-earned failure. They just have to happen. So, stop stockpiling and pushing around data that isn’t getting you anywhere. Decide first what you need to revolutionize, how you’re going to measure it, and how quickly you can fail.
When you need guidance on how to take that turn toward getting it right with data or setting up the first experiment, we can help you stand apart from the crowd.